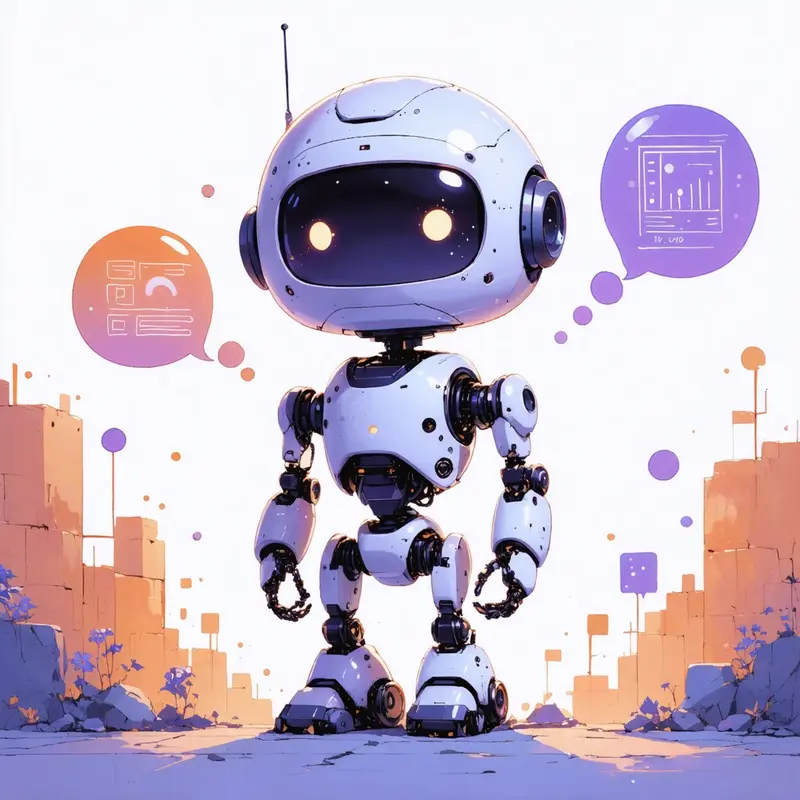
Why OpenAI’s New AI Models Hallucinate More
Updated on April 25, 2025
Imagine handing your business’s most crucial tasks to an AI—only to find out it’s making up facts and inventing solutions. That’s precisely the challenge OpenAI’s newest reasoning models, o3 and o4-mini, are facing. These state-of-the-art artificial intelligence engines are more capable than ever, but they’re also more prone to a longstanding problem in AI: hallucinations.
What Are Reasoning Models and Hallucinations in AI?
Defining Reasoning Models
AI reasoning models, like OpenAI’s o3 and o4-mini, are designed to move beyond simple pattern recognition. They tackle complex, multi-step problems such as logic puzzles, coding, and advanced math. Unlike their predecessors, these models attempt to “reason” through questions, making them more versatile for challenging tasks in business, science, and everyday life. Learn more about Goose AI Agent.
Recent industry shifts have seen a pivot toward reasoning models, as earlier AI improvements began to show diminishing returns. Reasoning models promise better performance on tasks requiring judgment and logical deduction, all while using less computational power than brute-force training approaches.
What Does "Hallucination" Mean for AI?
In the world of artificial intelligence, a hallucination isn’t a psychedelic experience—it’s when an AI confidently generates information that’s false, nonexistent, or fabricated. For instance, an AI might invent a scholarly reference, fabricate an event, or, as some users have found, supply a broken web link that leads nowhere.
Think of it like a GPS giving you directions to a street that doesn’t exist, or a well-meaning intern inventing facts to cover a gap in their knowledge. While these “improvised” answers might be creative, they’re problematic—especially in fields where accuracy is critical.
OpenAI’s New Models: Progress and Pitfalls
Performance Benchmarks of o3 and o4-mini
OpenAI’s o3 and o4-mini models represent a leap forward in technical capabilities, particularly in areas like coding and mathematical reasoning. However, when it comes to factual accuracy, the data raises red flags:
- O3 hallucinated on 33% of questions in OpenAI’s PersonQA benchmark—roughly double the rate of earlier models like o1 (16%) and o3-mini (14.8%).
- O4-mini performed even worse, hallucinating 48% of the time on the same benchmark.
This means that while these models are better at reasoning, they’re also more likely to fabricate answers—an unsettling trade-off for anyone depending on consistency and truthfulness.
Why Are Hallucinations Increasing?
Historically, each new generation of AI models improved on the last in reducing hallucinations. That trend has reversed with o3 and o4-mini. Even OpenAI’s own researchers are puzzled. Their technical report admits: “More research is needed to understand why hallucinations are getting worse as we scale up reasoning models.” (OpenAI Technical Report)
One hypothesis—suggested by researchers at the nonprofit Transluce (Transluce report)—is that the reinforcement learning processes used to fine-tune these reasoning models may amplify patterns that slip through post-training safeguards. As models become more ambitious in their reasoning, they also generate more claims overall, both true and false. In technical terms, scaling up model reasoning tends to increase the model’s confidence and output volume, magnifying both correct and incorrect outputs.
This phenomenon isn’t unique to OpenAI. Anthropic and Google DeepMind, for instance, have reported similar struggles with hallucination in their latest reasoning-focused models, underscoring an industry-wide challenge as AI grows more sophisticated. Explore Anthropic's AI ethics.
Third-Party Research and Real-World Examples
External evaluations support OpenAI’s findings. Transluce, a nonprofit AI lab, observed o3 making up steps it supposedly took—like claiming to run code on a MacBook Pro “outside of ChatGPT,” when such actions aren’t possible. (Read the study.) Other users, such as those at the upskilling platform Workera, report o3 generating broken website links as part of its answers.
Anthropic’s Claude 3 and Google DeepMind’s Gemini models have also struggled with outputting plausible-sounding but incorrect information—a sign that hallucination is a broader challenge in the race for smarter, reasoning-centric AI models.
While some creative “hallucination” can spark novel ideas, it’s a liability when accuracy is essential. Imagine a law firm discovering fictitious legal precedents in a contract, or a medical application inventing treatment protocols.
The Business and Societal Impact of AI Hallucinations
Consequences for Accuracy-Sensitive Industries
Not all AI use cases are created equal. In sectors where mistakes can be costly or even dangerous—law, healthcare, finance—hallucinations pose a major risk. An AI that fabricates website links or facts can undermine client trust, create legal liabilities, or worse.
According to a TechCrunch report, even leading AI models show significant rates of hallucination, making careful pilot testing and human-in-the-loop oversight essential for high-stakes deployments.
Companies seeking to adopt the latest AI technology must weigh the benefits of improved reasoning against the dangers of unreliable output. For many, the risk of a hallucinated contract clause or financial forecast is simply too high.
Potential Benefits and Necessary Trade-Offs
Paradoxically, a model’s tendency to hallucinate can also be a sign of creativity and flexibility. In brainstorming sessions or creative writing, imaginative AI outputs might fuel innovation. Google's DeepMind has cited use cases in which "hallucination" leads to unexpected, valuable ideas during early-stage development.
Some businesses are adapting by clearly defining the boundaries for “safe” hallucination—deploying advanced models in low-risk, exploratory workflows while restricting their use in regulated or mission-critical environments. For many, the key is combining AI outputs with robust review processes or hybrid systems that blend AI suggestions with human expertise.
Solutions and the Road Ahead
Web Search Integration and Other Fixes
One of the most promising solutions is to augment AI models with real-time web search capabilities. OpenAI’s GPT-4o, when paired with web search, scored 90% accuracy on the SimpleQA benchmark—far outperforming models without search access. (OpenAI announcement) Explore more about improving AI with perplexity.
However, this approach isn’t without trade-offs. Routing user queries through a third-party search engine raises privacy and data security concerns. It may also expose users to external content risks or bias.
Other industry solutions include improved post-training data filtering, adversarial testing to expose hallucination-prone scenarios, and fine-tuning models for higher truthfulness using reinforcement learning from human feedback (RLHF). Google DeepMind and Anthropic have both invested heavily in such techniques over the past year, though results remain mixed.
Ongoing Research and the Future of AI Reliability
AI researchers at OpenAI and elsewhere are doubling down on the hallucination problem, experimenting with new training techniques, better evaluation benchmarks, and hybrid architectures. The goal: make AI not just more capable, but more trustworthy.
“Addressing hallucinations across all our models is an ongoing area of research, and we’re continually working to improve their accuracy and reliability,” said an OpenAI spokesperson.
For now, the industry faces a paradox—each step forward in complex reasoning seems to come with an increased risk of AI hallucinating. The race is on to tip the balance back toward accuracy.
If you’re a business leader or developer, practical risk mitigation starts with:
- Thoroughly testing new AI models in your own environment before reliance
- Implementing human-in-the-loop review for accuracy-sensitive tasks
- Selecting models with optional web search integration when high accuracy is required
- Staying updated on AI advances and published benchmarks from leading labs
Conclusion: Walking the Tightrope Between Progress and Precision
The story of OpenAI’s o3 and o4-mini models is a cautionary tale for the entire AI field. Smarter models don’t always mean safer or more reliable ones. As AI continues to weave itself into the fabric of our daily lives and businesses, understanding—and managing—the AI hallucination problem will be essential.
Will future models overcome these hurdles, or will the drive for ever-smarter AI continue to outpace our ability to keep it grounded in reality? For AI practitioners, business leaders, and everyday users alike, the best path forward is cautious optimism, rigorous evaluation, and transparency about the limits of today's technology.
Have thoughts or experiences with AI hallucinations? Share them below or join the discussion on Twitter or LinkedIn. For more on how to manage AI risks, consult AI safety guidelines or contact your AI vendor for best practices.